Exploring the power of synthetic data in the era of advanced analytics
Explore synthetic data's role in AI, privacy, and research. Learn how it powers advanced analytics across industries in this article.
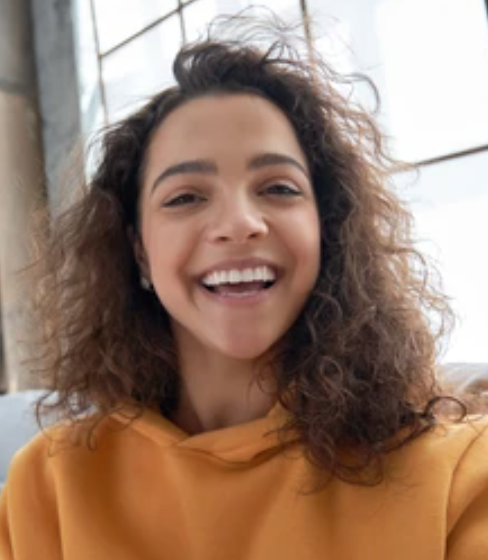
Explore synthetic data's role in AI, privacy, and research. Learn how it powers advanced analytics across industries in this article.
In today's data-driven world, the demand for large and diverse datasets is ever-increasing. However, acquiring and using real-world data for various purposes can be challenging due to privacy concerns, limited availability, or cost restrictions. Enter synthetic data – a game-changing solution that offers a realistic alternative. In this article, we delve into the fascinating world of synthetic data, its benefits, and its applications across industries. From machine learning and AI development to data privacy and research, synthetic data has emerged as a valuable asset for organizations seeking to unlock the potential of advanced analytics.
Synthetic data refers to artificially generated data that mimics the statistical properties and patterns of real-world data. It is created using various techniques, including generative models, simulations, and algorithms. By replicating the characteristics of actual data, synthetic data allows researchers, data scientists, and businesses to perform robust analyses and model training without compromising privacy or dealing with limitations associated with real data.
Synthetic data can be customized to match specific scenarios, incorporating varying levels of complexity and diversity. It retains essential statistical properties such as distribution, correlation, and variability, enabling accurate simulations and analysis. This artificial data offers several advantages, including scalability, cost-effectiveness, and reduced data acquisition time.
With increasing regulations and privacy concerns, synthetic data provides a viable solution for safeguarding sensitive information. By generating synthetic counterparts, organizations can ensure data privacy without compromising the utility of the data. Synthetic data eliminates the risk of reidentification, ensuring compliance with data protection regulations such as GDPR and HIPAA.
Synthetic data facilitates rapid and iterative development of machine learning models. By generating diverse datasets with known ground truths, data scientists can efficiently train, validate, and fine-tune models without the limitations of real data availability. Synthetic data also allows for stress-testing and scenario simulations, enabling robust model performance evaluation.
Data augmentation is a crucial technique for enhancing model performance and generalization. Synthetic data complements traditional data augmentation methods by introducing additional variations, edge cases, and rare scenarios that might be underrepresented in real-world data. This process enriches the training data, improving model resilience and adaptability.
Synthetic data offers the opportunity to create balanced datasets, mitigating biases that may exist in real data. By carefully designing and generating synthetic samples, organizations can ensure fairness in AI algorithms and prevent biased decision-making.
Synthetic data enables organizations to share valuable insights and collaborate without compromising privacy or proprietary information. By generating synthetic replicas of sensitive data, organizations can foster data-driven collaborations, research, and innovation while protecting sensitive information.
While synthetic data presents numerous advantages, ensuring its quality and validity is paramount. Generating realistic synthetic data requires careful consideration of underlying patterns, distributions, and correlations present in the real data. Proper validation and evaluation processes should be in place to ensure that synthetic data accurately represents the target domain.
Additionally, understanding the limitations of synthetic data is essential. While it can replicate statistical properties, synthetic data may not capture the nuances or complexities present in the real world. Close collaboration between domain experts and data scientists is crucial to validate the usability of synthetic data and assess its applicability for specific use cases.
As the demand for data-driven insights continues to grow, synthetic data emerges as a valuable asset in the realm of advanced analytics. Its ability to mimic real data while preserving privacy and addressing limitations opens up new possibilities for research, model development, and data sharing. Synthetic data has the potential to revolutionize industries, empowering organizations to make informed decisions and foster innovation. By leveraging this innovative approach, businesses can unlock the power of synthetic data to drive advancements in machine learning, AI, and beyond.
Contactez nos experts pour des solutions innovantes et personnalisées
Tester la plateforme gratuitementNous contacter15 jours d'essai
Pas de carte de crédit
Annulez à tout moment